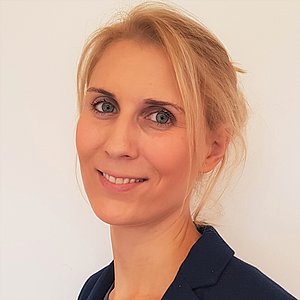
Prof. Dr. Marina Höhne
Abteilung: Data Science in Bioeconomy
Mitarbeit in Forschungsprogrammen
Mitwirkung in Gremien
- Climate Change Center Berlin Brandenburg
- ELLIS Gesellschaft, the European Laboratory for Learning and Intelligent Systems
- Berlin AI Competence Center BIFOLD, the Berlin Institute for the Foundations of Learning and Data
Projekte
- SPIN-FERT – Innovative practices, tools and products to boost soil fertility and peat substitution in horticultural crops The main objective of SPIN-FERT is to integrate optimised and validated innovations in soil management practices and improve pea…
- DCropS4OneHealth-2 – Diversifying cropping systems for the One Health of soils, plants and humans Die Diversifizierung von Pflanzenbausystemen in Verbindung mit der Senkung des Einsatzes von Agrochemikalien und einer Erhöhung der Landschaftskomplexit…
- REFRAME – Erforschung und Erweiterung von Computer Vision Foundation Models (ExploRing and Expanding the FrontierRs of FoundAtion ModEls) Im Bereich der Computer Vision haben Vision Foundation Models (VFM) neue Maßstäbe in visuellen Aufgaben zum Vers…
- Joint Lab KI & DS – Joint Lab Künstliche Intelligenz & Data Science Im Rahmen des Joint Lab bilden die Universität Osnabrück und das ATB gemeinsam Doktorandinnen und Doktoranden an der Schnittstelle von Agrarwissenschaft und Künstlicher Intelligenz a…
- Explaining 4.0 – Künstliche Intelligenz - Transparenz und Effizienz Das Ziel des Projektes Explaining 4.0 ist die Entwicklung von Methoden, die einen signifikanten Beitrag zu einem ganzheitlichen -globalen- Verständnis von KI-Modellen leisten. Dabei …
- DCropS4OneHealth-1 – Diversifying cropping systems for the One Health of soils, plants and humans; BiodivGesundheit: Diversifizierung von Pflanzenbausystemen für die gemeinsame Gesundheit von Böden, Pflanzen und Menschen Die Diversifizierung von Pfl…
- XAI-Mobil – Towards Reliable Artificial Intelligence for Explainable, Interactive and Self-evolving Systems Projekt im Rahmen des Mobilitätsförderprogramms des Chinesisch-Deutschen Zentrum für Wissenschaftsförderung mit Forschern der Sun Yat-sen Univ…
Veröffentlichungen
- Babor, M.; Liu, S.; Arefi, A.; Olszewska-Widdrat, A.; Sturm, B.; Venus, J.; Höhne, M. (2024): Domain-Invariant Monitoring for Lactic Acid Production: Transfer Learning from Glucose to Bio-Waste Using Machine Learning Interpretation. Bioresource Technology. : p. 1-23. Online: Preprint: http://dx.doi.org/10.2139/ssrn.5012080
- Arefi, A.; Sturm, B.; Babor, M.; Horf, M.; Hoffmann, T.; Höhne, M.; Friedrich, K.; Schroedter, L.; Venus, J.; Olszewska-Widdrat, A. (2024): Digital model of biochemical reactions in lactic acid bacterial fermentation of simple glucose and biowaste substrates. Heliyon. (19): p. 38791. Online: https://doi.org/10.1016/j.heliyon.2024.e38791
- Olszewska-Widdrat, A.; Babor, M.; Höhne, M.; Alexandri, M.; López Gómez, J.; Venus, J. (2024): A mathematical model-based evaluation of yeast extract’s effects on microbial growth and substrate consumption for lactic acid production by Bacillus coagulans. Process Biochemistry. (November): p. 304-315. Online: https://doi.org/10.1016/j.procbio.2024.07.017
- Bommer, P.; Kretschmer, M.; Hedström, A.; Bareeva, D.; Höhne, M. (2024): Finding the right XAI Method - A Guide for the Evaluation and Ranking of Explainable AI Methods in Climate Science. Artificial Intelligence for the Earth Systems (AIES). (3): p. 1-26. Online: https://doi.org/10.1175/AIES-D-23-0074.1
- Bareeva, D.; Höhne, M.; Warnecke, A.; Pirch, L.; Müller, K.; Rieck, K.; Bykov, K. (2024): Manipulating Feature Visualizations with Gradient Slingshots. arXiv. : p. 1-19. Online: https://doi.org/10.48550/arXiv.2401.06122
- Hedström, A.; Weber, L.; Lapuschkin, S.; Höhne, M. (2024): Sanity Checks Revisited: An Exploration to Repair the Model Parameter Randomisation Test. arXiv. : p. 1-19. Online: https://arxiv.org/abs/2401.06465
- Gautam, S.; Boubekki, A.; Höhne, M.; Kampffmeyer, M. (2023): Prototypical Self-Explainable Models Without Re-training. arXiv. : p. 1-25. Online: https://arxiv.org/abs/2312.07822
- Grinwald, D.; Bykov, K.; Nakajima, S.; Höhne, M. (2023): Visualizing the Diversity of Representations Learned by Bayesian Neural Networks. Transactions on Machine Learning Research. (11): p. 1-25. Online: https://openreview.net/pdf?id=ZSxvyWrX6k
- Hanfeld, P.; Wahba, K.; Höhne, M.; Bussmann, M.; Hönig, W. (2023): Kidnapping Deep Learning-based Multirotors using Optimized Flying Adversarial Patches. arXiv. : p. 1-7. Online: https://doi.org/10.48550/arXiv.2308.00344
- Hanfeld, P.; Höhne, M.; Bussmann, M.; Hönig, W. (2023): Flying Adversarial Patches: Manipulating the Behavior of Deep Learning-based Autonomous Multirotors. arXiv. : p. 1-6. Online: https://doi.org/10.48550/arXiv.2305.12859
Weitere Veröffentlichungen
- Gautam, S., Höhne, M. M.-C., Hansen, S., Jenssen, R., & Kampffmeyer, M. (2022). Demonstrating The Risk of Imbalanced Datasets in Chest X-ray Image-based Diagnostics by Prototypical Relevance Propagation. In 2022 IEEE 19th International Symposium on Biomedical Imaging (ISBI) (pp. 1-5). IEEE
- Hedström, A., Weber, L., Bareeva, D., Motzkus, F., Samek, W., Lapuschkin, S., and Höhne, M. M.-C. (2022). Quantus: an explainable AI toolkit for responsible evaluation of neural network explanations. arXiv preprint arXiv:2202.06861
- Mieth, B., Rozier, A., Rodriguez, J. A., Höhne, M.M.-C., Görnitz, N., & Müller, K. R. (2021): DeepCOMBI: explainable artificial intelligence for the analysis and discovery in genome-wide association studies. NAR Genomics and Bioinformatics, 3(3), lqab065
- Bykov, K., Deb, M., Grinwald, D., Müller, K.R. and Höhne, M.M.-C., 2022. DORA: Exploring outlier representations in Deep Neural Networks. arXiv preprint arXiv: 2206.04530
- Bykov, K., Hedström, A., Nakajima, S., and Höhne, M.M.-C. (2021): NoiseGrad: enhancing explanations by introducing stochasticity to model weights. In Proceedings of the AAAI Conference on Artificial Intelligence (Vol. 36, No. 6, pp. 6132-6140)
- Bykov, K., Höhne, M.M.-C., Creosteanu, A., Müller, K.-R., Klauschen, F., Nakajima, S., & Kloft, M. (2021). Explaining bayesian neural networks. arXiv preprint arXiv:2108.10346
- Mieth, B., Hockley, J. R., Görnitz, N., Vidovic, M.M.-C., Müller, K. R., Gutteridge, A., and Ziemek, D. (2019): Using transfer learning from prior reference knowledge to improve the clustering of single-cell RNA-Seq data. Scientific reports, 9(1), 1-14
- Vidovic, M.M.-C., Kloft M., Müller K.-R., and Görnitz N., 2017. ML2motif – Reliable extraction of discriminative sequence motifs from learning machines. PloS one 12.3, e0174392
- Vidovic M.M.-C., Görnitz N., Müller K.-R., and Kloft M., 2016. Feature importance measure for non-linear learning algorithms. arXiv preprint arXiv:1611.07567
- Vidovic, M.M.-C., Hwang H.J., Amsüss S., Hahne J.M., Farina D., and Müller K.-R. (2016): Improving the robustness of myoelectric pattern recognition for upper limb prostheses by covariate shift adaptation. IEEE Transactions on Neural Systems and Rehabilitation Engineering, 24.9, 961-970
- Vidovic, M.M.-C., Görnitz N., Müller K.-R., Rätsch G., and Kloft M. (2015): SVM2Motif Reconstructing Overlapping DNA Sequence Motifs by Mimicking an SVM Predictor. PloS one 10.12, e0144782