Research for animal welfare and environmental health
Concepts of livestock farming should incorporate the three pillars of sustainability: environment, society and economy. While there is a growing desire in society for improved animal welfare and enhanced environmental protection, the need for economic survival on the part of farmers must be taken into account at the same time. This requires innovative processes to find their way into practice.
We therefore focus our application-oriented basic research in animal husbandry in line with the One Health approach on improving animal welfare, on husbandry environment, animal and environmental protection as well as on the competitivity of agriculture. Our goals are: objective animal welfare standards, flexible and regionally adapted concepts to solve environmental conflicts, a transparent animal husbandry, consumer acceptance and regional added value.
Our research in this program area is particularly concerned with digital methods for monitoring animal welfare, housing climate and emissions, as well as the evaluation of animal housing systems, especially in open-ventilated and novel housing systems. We develop fundamental knowledge on the generation and dispersion of gaseous emissions and pathogen-laden particles as well as on the ways to minimize the risk of spreading multi-resistant pathogens.
The ATB boundary layer wind tunnel offers extensive possibilities for the investigation of airflow and dispersion processes around agricultural buildings as well as airflow and ventilation processes within the buildings. The detailed understanding at process level feeds into modeling approaches with the goal of estimating emissions from new housing systems and finding opportunities for low emissions in animal-friendly housing.
We consider animal husbandry as a part of circular agricultural systems and integrate our research into the holistic, bioeconomic concept of the Leibniz Innovation Farm, which is currently being established in Groß Kreutz.
We develop and test the use of non-invasive sensors for the animal-specific recording of stress and health. In this context, we analyze animal-individual indicators such as activity, respiration rate, body temperature and performance together with stable climatic parameters in different animal species and husbandry systems, increasingly by using data science, artificial intelligence and sensor fusion. Our aim is to develop automated decision support and control units based on animal-related indicators. Early warning systems are to support farmers in sustainably improving animal health and welfare.
Barn climate and emissions
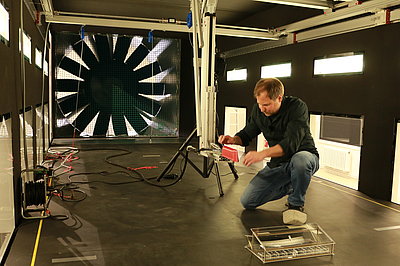
We are developing algorithms to efficiently measure, characterize, and model the dynamics of pollutants that are harmful to the environment, climate, and/or health, and to mitigate infection and antimicrobial resistance (AMR). Within our three-pillar model, we combine measurements in different real-world husbandry systems with physical and mathematical modeling to form an overall system. The solutions developed here are intended to strengthen the resilience of farms and contribute to energy efficiency, moving away from fossil raw materials and reducing emissions.
Containing infections and antibiotic resistance
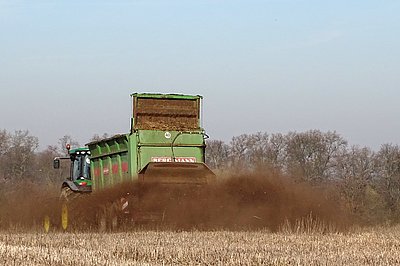
Our research follows the One Health approach and aims at prevention and control measures against the spread of zoonoses, infectious diseases and antibiotic resistance in the livestock sector. For example, we are investigating the influence of feed additives and improved hygiene measures in pig farming, the effectiveness of different farm manure treatments and parameter variations on the reduction of AMR bacteria in poultry farming, and are developing an early warning model for mastitis in dairy farming.
Assessment of animal husbandry systems
Our objective for a sustainable livestock production is to close material cycles, to improve nitrogen efficiency, to reduce GHG emissions and to develop individual, regional and flexible concepts for circular agriculture and food systems. To do this, we use methods of modeling, systems analysis, and multi-criteria evaluation, including quantification of trade-offs and synergies.
The results are fed into operational decision support systems and proposals for stock improvement as well as into emission models for the analysis of country-specific scenarios and inventories. We also collaborate closely with international bodies at IPCC, UNECE and FAO in this area.
To the team of the programme area
Research projects
-
The AirBarn project aims to analyse aerosols in and from animal stalls as a potential source of danger for airborne pathogens. The scientific study is taking place as part of the Leibniz research network INFECTIONS, whic…
-
Livestock farming is a key sector that involves 40 % of the total agricultural activity in Europe. representing a total value for products equal to 170 billion. However, there is an increasing concern due to livestock f…
-
The AMRAfrika project aims to create a comprehensive assessment model for antimicrobial use (AMU) and resistance (AMR) in cattle production systems. It combines participatory data gathering methods with digital data mana…
-
This pilot study will collect first-hand data on antibiotic use in cattle farming and the prevalence of antibiotic resistance in animal food (ASF), humans and the environment in South Kivu province in the eastern Democra…
-
In the EmiMod joint project, methods for determining emissions from diffuse area sources (e.g. freely ventilated pig and cattle stalls with runs/running yards and other external emission sources such as slurry tanks) are…
More projects within the programme area
Publications of the programme
- Cantillon, M.; Hennessy, T.; Amon, B.; Dragoni, F.; OBrien, D. (2024): Mitigation of gaseous emissions from dairy livestock: A farm-level method to examine the financial implications. Journal of Environmental Management. (14 February 2024): p. 119904. Online: https://doi.org/10.1016/j.jenvman.2023.119904 1.0
- Yuan, H.; Du, L.; Cao, Y.; Tu, S.; Liu, D.; Yi, Q.; Luo, W.; Gao, H.; Zhang, J.; Liao, F.; Fu, H.; Liao, Y. (2024): Understanding the role of ?-? stacking from red carbon for selective, high stable and anti-external interference detecting ferric ions and cellular imaging. Microchemical Journal. : p. 0. Online: https://doi.org/10.1016/j.microc.2024.110416 1.0
- Diaz de Otálora Aguirre, X.; Amon, B.; Balaine, L.; Dragoni, F.; Estellés, F.; Ragaglini, G.; Kieronczyk, M.; Jorgensen, G.; Del Prado, A. (2024): Influence of farm diversity on nitrogen and greenhouse gas emission sources from key European dairy cattle systems: A step towards emission mitigation and circularity. Agricultural Systems. (April): p. 103902. Online: https://doi.org/10.1016/j.agsy.2024.103902 1.0
- Petersen, S.; Ma, C.; Hilgert, J.; Mjöfors, K.; Sefeedpari, P.; Amon, B.; Aarnink, A.; Franko, B.; Dragoni, F.; Groenestein, K.; Gyldenkrne, S.; Herrmann, C.; Hutchings, N.; Kristensen, S.; Liu, J.; Olesen, J.; Rodhe, L. (2024): In-vitro method and model to estimate methane emissions from liquid manure management on pig and dairy farms in four countries. Journal of Environmental Management. (Februar): p. 120233. Online: https://doi.org/10.1016/j.jenvman.2024.120233 1.0
- Kashongwe, O.; Kabelitz, T.; Ammon, C.; Amon, T.; Doherr, M.; Amon, B. (2024): Influence of Preprocessing Methods of Automated Milking Systems Data on the Prediction of Mastitis with Machine Learning Models. AgriEngineering. (6): p. 3427-3442. Online: https://doi.org/10.3390/agriengineering6030195 1.0
- Ouatahar, L.; Bannink, A.; Zentek, J.; Amon, T.; Deng, J.; Hempel, S.; Janke, D.; Beukes, P.; Van der Weerden, T.; Krol, D.; Lanigan, G.; Amon, B. (2024): An integral assessment of the impact of diet and manure management on whole-farm greenhouse gas and nitrogen emissions in dairy cattle production systems using process-based models. Waste Management. (October): p. 79-90. Online: https://doi.org/10.1016/j.wasman.2024.07.007 1.0
- Thomas, C.; Idler, C.; Ammon, C.; Amon, T. (2024): Applied Research Note: Survival of Escherichia coli and temperature development during composting of chicken manure with a typically low carbon/nitrogen ratio and moisture content. Journal of Applied Poultry Research. : p. 0. Online: https://doi.org/10.1016/j.japr.2024.100402 1.0
- Kurras, F.; Jakob, M. (2024): Smart dairy farming-The potential of the automatic monitoring of dairy cows’ behaviour using a 360-degree camera. animals. (4): p. 640. Online: https://doi.org/10.3390/ani14040640 1.0
- Amenu, K.; Shibiru, A.; Kumbe, A.; Konyole, S.; Jaleta, M.; Tiki, W. (2024): Perceptions of rural and urban residents in Borana pastoral and agro-pastoral areas in Ethiopia related to milk adulteration. BMC Nutrition. (10): p. 0. Online: https://doi.org/10.1186/s40795-024-00876-4 1.0
- Fromm, K.; Heinicke, J.; Ammon, C.; Amon, T.; Hoffmann, G. (2024): Performance of a UHF RFID Detection System to Assess Activity Levels and Lying Behaviour in Fattening Bulls. AgriEngineering. (6): p. 1886-1897. Online: https://doi.org/10.3390/ agriengineering6020110 1.0
More publications of the programme area